NIST released the NIST AI RMF about a year ago.
Key Takeaways: One year later, the NIST AI Risk Management Framework has some serious shortcomings, in this article we will discuss how to solve them.
ABC published a Q&A with Elham Tabassi who provided leadership on developing and releasing that framework.
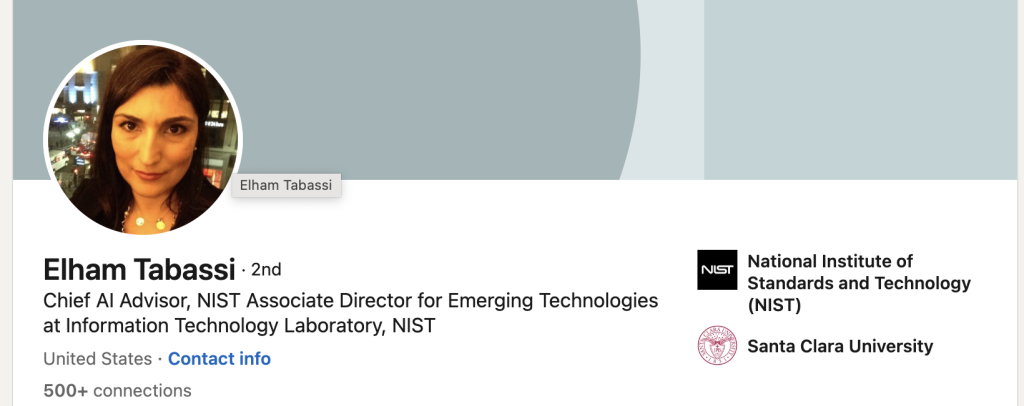
She re-introduced the framework at the IAPP Privacy, Security, and Risk conference in San Diego over the summer of 2023. It was there, that she was careful to emphasize that the framework was mostly a list of suggestions.
It was clear that NIST was hedging in that session. An engineer from Intuit cleverly posed this question, “How durable is the NIST framework?” Tabassi, sheepishly admitted, “Not very.” What this means essentially is that the NIST AI framework was relevant perhaps at a snapshot of time, but will quickly become obsolete due to the rapid advances in the AI they are seeking to govern. – Forest Global Training Partners has developed a proposed solution to this very issue and we are offering to contribute to NIST for their next version of the RMF to solve that problem precisely.
The NIST AI RMF is currently just a “College Try” to solve a very complex engineering problem. Some of the outputs of the NIST AI RMF are suspect, while others show that the NIST AI RMF included discriminatory assumptions.
If you study the framework in-depth, it is clear that the NIST framing of “Bias” only serves to introduce that committee’s preferred biases over other biases that they find abhorrent.
Their solution to bias was to create guidelines. These guidelines suggest AI Governance panels should implement social justice in the form of AI training input data, as well as AI output behavior.
Social justice is a political worldview that is currently popular, but is not something that should be a guiding principal of machine learning. Rather, it should be a data point, after all, won’t humans some day rely on AI to solve or transcend the very political problems that we have created for ourselves?
To introduce one type of bias, and then claim that is the solution to bias, is intellectually dishonest. Humans are inherently biased.
If we look to AI to solve problems, and pretend that we have successfully caged one aspect of machine learning with laws, regulations, or frameworks, that is a foolish conclusion.
So, why then, do we even attempt AI Governance, and are frameworks altogether useless?
Let’s start by defining a framework. What is a framework?
If one thinks of a framework in terms of the analogy of physical architecture, such as the skeletal framing of a house or a building, that is a decent starting point to understand what we mean by framework.
What does a framework do? In the physical world, a framework provides a multi-dimensional structure to enable further development of a thing such as a building. You have a floor, exterior walls, interior walls, closets, bathrooms, and office space. What you have is three dimensional, and this starts to solve the problem of providing shelter.
In the systems engineering world, we are constructing frameworks on a theoretical level. Because the problems we regularly solve are abstract, complex, and multi-dimensional.
Frameworks serve to provide structure. The best frameworks, in system engineering, provide not only structure, but a basis for understanding. They provide a blueprint, as well as a set of concrete steps to build the framework. This enables a team to put in motion a framework performing its function; framing a complex system.
The NIST AI RMF lacks the concrete set of steps that an AI Risk framework should have. In addition, Elham Tabassi admitted, instead, that the NIST AI RMF is a set of suggestions; rather than actionable steps.
It is very difficult to take a set of suggestions seriously when it comes to something as important as AI.
It is also difficult to find the will-power of Engineering, Legal, and Operational teams to accomplish their objective. Will-power and the coordination of these teams are required to steer the ship to a successful outcome. A poorly constructed framework is a formidable obstacle to reaching that outcome.
Still, the NIST AI RMF was the first framework to the table, and as such, it has had the longest time to chum the waters and kick up relevant discussions around AI Governance. Being the first at anything is difficult. The NIST AI RMF will likely undergo revisions and other experts will likely solve some of the most urgent problems that it poses.
Until then, corporations, government entities, and individuals using AI have to determine if they will try to implement the NIST framework as it stands.
Here are the current problems and solutions with the NIST AI RMF:
Problem Number 1: The NIST AI RMF is not durable. This means it can quickly go out of date, and would be difficult to implement.
Solution 1: Carefully examine the NIST AI RMF and determine if it is the correct framework to adopt. If it is, then task your organization’s AI Governance team with solving for durability. This means that the team needs to protect against obsolescence, and future-proof the solution, by making it “evergreen”.
==
Problem 2: The NIST AI RMF pretends to solve bias while including it’s own preferred bias, which happens to be discriminatory in nature.
Solution 2: Stop trying to have a scientific tool, such as AI, adopt your favored/preferred political philosophy. Recognize that AI is still a machine, and introducing one bias to replace another bias, which will only result in a loss of trust for your AI model and AI implementation. Attempting to have AI behave in a discriminatory way, is likely to invite legal repercussions.
==
Problem 3: The NIST AI RMF lacks a concrete set of steps, which makes it very difficult to get started. In addition, the lack of a clear entry point, also increases the learning curve to implement the NIST AI RMF.
Solution 3: Develop an AI Governance team or AI Risk Management team. Have that team set out with developing their work rules. These rules are a prerequisite to begin the process of implementing the framework. Ensure that the team has members who review the accuracy, safety, risks, and outputs. Also, gather and track relevant metrics for that team to measure progress. These measurements will prove critical to a successful implementation of the NIST or any other framework.
==
Forest Global Training Partners is enthusiastic to help your organization adopt AI while reducing risks.
We support your organization’s AI Adoption efforts, while keeping watch of the potential harmful results to your company’s reputation, integrity and bottom line.
Here is the Q&A article with ABC and Elham Tabassi:

Best wishes on your AI Journey,
- Rory Siems President and CEO, Forest Global Training Partners