Just trying to understand what the average person does not know about Rail Cargo sends me on a research task that is already Flirting with Disaster. While I am by no means an industry expert, I do have a handful of family members that are either currently working for, or recently retired from the Railroad Industry.
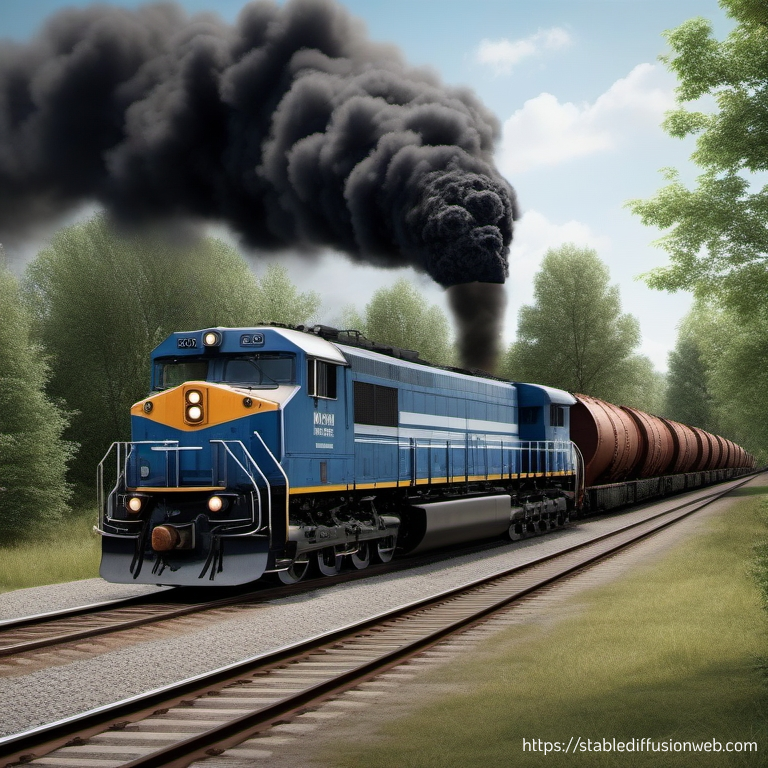
As a matter of fact, my great grandfather worked for a railroad in South Dakota, perhaps it was that, and growing up around electric trains, then later taking a commuter train to work on a daily basis. I have had the opportunity to look at trains up close and observe Rail Operations from an up-close perspective.
When I set out to establish a company that manages risk for Artificial Intelligence in various different industries, Rail Cargo was a must to include in considering those industries that would likely have both an interest in implementing AI quite broadly and also would have quite a large exposure to risk.
When thinking of risks in the Rail Industry, most people might tend to think of something like the high-profile derailments that we hear about in the news.
As it turns out, there are thousands of derailments that happen regularly especially in the context of rail yards. The Rail Yard derailments are technically derailments, even though a particular wheel of a particular car just came off, and requires re-setting. Even though such incidents are technically a derailment, they do not rise to the level of what the people of East Palestine Illinois endured when the Norfolk Southern Line had a catastrophic derailment near that town. You can read what people (and bots) are debating on that topic on reddit, so I won’t waste time re-visiting it here.
Aside from derailments, collisions at crossings may be a much more dangerous risk with Railroads, and Rail Cargo Transport, because there are tens of thousands of crossings (one industry insider put that number at around 40,000) on just the tracks that they own! So collisions at rail crossings seems to remain a more common, and perhaps a more statistically relevant, (more dangerous from a statistical perspective).
The Rail Industry as a whole brings some formidable risks to the table because of many factors that include: the power of locomotives, the size and speed of trains, track maintenance and upkeep, locomotive and rail car maintenance, and the transport of dangerous, toxic chemicals and materials.
Much like the Airline Industry has the FAA, which covers not only the airlines, but more broadly, any aircraft that files, so to, the Rail Industry has the FRA The Federal Railroad Administration. To be sure, the FRA has at the top of their duty things like Safety and Risk Management, which are promulgated in the form of regulations. The FRA regulations dictate everything one might imagine with regard to the safe operation of trains.
Let’s take a look at some possible use cases that ChatGPT thinks it knows about Rail Cargo for example and perhaps learn a bit more about creating some hypotheses around these use cases and determine those individual risks.
Possible AI Use Cases for the Railroad Industry
Here are the Use Cases: first we have, Freight Routing and Scheduling, the proposition of using AI in this case, perhaps in processing data related to operations looks promising! So the Rail Industry generates metric tons of data every single day, synthesizing this data with AI performing some statistical analysis would greatly assist in this regard.
Things like, crew rest schedules, crew bids, scheduling, relief, trips and repositioning, hundreds of thousands of data points just around the train crews around the system. The crew data informs things like dispatching. Dispatching and Routing perhaps two sides of the same coin.
Here again we can safely presume that there is a vast amount of data to process to enable any Rail company. For routing and scheduling purposes, the Risks that are there are the same that would be present either during normal operations or AI-Enhanced operations. Those risks are likely contextually lower than other use cases, but they are definitely not zero. There is still a chance that AI could magnify a risk that while not directly introduced by AI, was somehow made worse by AI.
This opinion comes from performing years of root cause analysis and having to troubleshoot disaster outcomes across complex multidimensional systems. The Rail Transport ecosystem, is one such complex multidimensional system.
The next use case is “Cargo Security and Monitoring”, AI can certainly assist or enhance, security especially along many miles of track, at secured facilities and by scanning codes on cargo, rail cars, and on shipping containers. The probability that AI is used in these roles, is most likely a matter of when not if. The question remains, what plan is in place in case the AI performing one of these critical functions provides an adverse reaction, or if it actually misinterprets its training functions and that results in a completely unwanted outcome?
The next Use Case is Maintenance Planning, Track Maintenance, Rail Yard Maintenance, Rail Car Maintenance, Locomotive Maintenance, and every moving part in the railroad industry requires regular maintenance and upkeep. It’s most likely that this maintenance is conducted on a regular schedule, and that schedule is it’s own logistical challenge, because maintenance crews require deployment, and locomotives need to be present at their required maintenance hub on a required date, not only for upkeep, but for compliance with FRA regulations.
Maintenance requirements, activities, and certification generate a ton of data in the Railroad industry. This is a task currently managed by software, ripe for AI statistical analysis, cognitive AI, and predictive analysis and process improvement. The risk here would be to become overly dependent on the improvements that AI introduces and over time lose sight of how to manage the system before the AI Era. So the AI Risk here increases over time as dependence on AI to realize these improvements increases. Here a disaster recovery, fall back plan, and manual scheduling capabilities must be maintained in order to decrease any critical dependencies that may, in the future grind things to a halt.
Another intriguing use case is Inventory Tracking and Management, what I think ChatGPT was trying to suss out is more the idea of Logistics, because Railroad Inventory, from my interpretation is most likely covered by dispatch, but perhaps an industry expert could weigh in here.
Finally, the last suggested use case for AI in the Railroad Industry is “Efficiency and Performance Optimization” I think this is a massive opportunity for AI Enablement in the rail cargo industry, and this use case I believe is one that is relatively low risk. I don’t see that AI is going to present significant risks for business optimization cases if the AI is narrow enough to produce short term gains, and at the same time, the business strategy protects from long term over-dependence on AI.
What’s next? I am keen to hear the opinions of industry experts who currently see AI Use Cases for the Rail Industry from the inside and might benefit from this type of analysis to uncover any possible hidden risks of AI that they may not have contemplated. If you are one such person, please leave a comment or feel free to reach out.